Water Quality Assessment of Dug Wells in Lagos Island, Southwestern Nigeria
Keywords:
Shallow wells, water, quality, Lagos IslandAbstract
Communication in Physical Sciences 2019, 4(2):110-117
Received 20 November 2019/Accepted 20 December 2019
Several evidences abound that shallow wells can constitute public health problems if its water is incidentally contaminated. Consequently, physicochemical parameters and metal concentration of groundwater samples from Lagos Island were analysed and compared with WHO (2011) standards. Water samples were taken from dug wells across four clusters within Lagos Island in Nigeria. Standard (AOAC) methods were adopted in the analysis of the physicochemical parameters while heavy metals ions determination was done using Atomic Absorption Spectrophotometer. The results obtained revealed noticeable discrepancy in the levels of some physicochemical properties and metal ion concentration in the water samples when compared with WHO (2011) standards. Though most physicochemical parameters were within safe limits, hardness, conductivity, concentrations of lead, zinc, calcium, manganese and potassium exceeded their permissible limits. Decreasing order for the concentration of the metal ions was Ca ˃ Mg ˃ K ˃ Zn ˃ Fe ˃ Cu ˃ Mn ˃ Pb ˃ Ni ˃ Cd. From the results and findings of the study, it is proposed that if mitigating measures are not implemented, well water within Lagos Island may constitute future environmental threat.
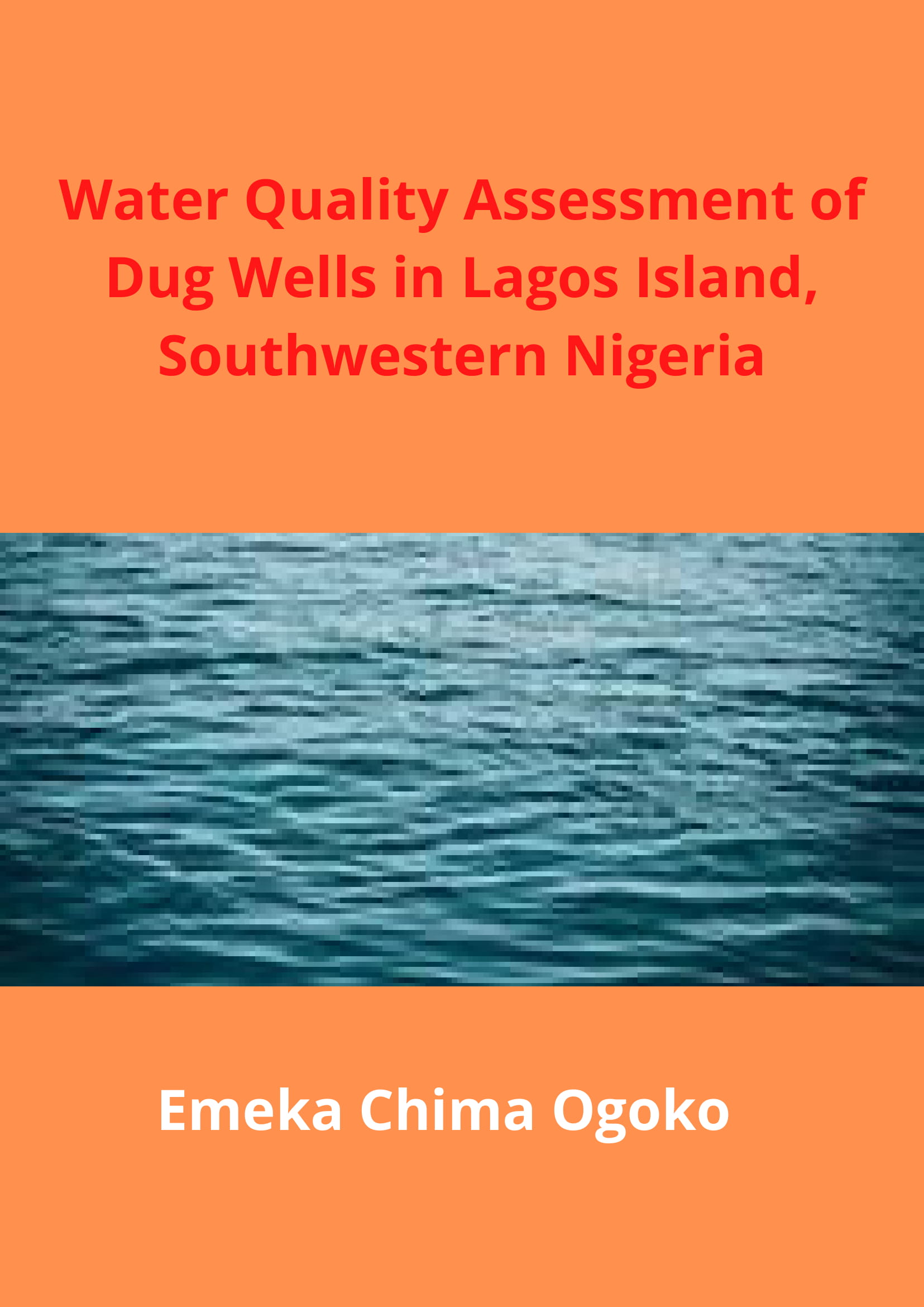
Downloads
Published
Issue
Section
Similar Articles
- Ajogwu Cordelia Odinaka, Aaron Auduson, Tope Alege, Yusuf Odunsanwo, Formation Evaluation Using Integrated Petrophysical Data Analysis of Maboro Field Niger Delta Sedimentary Basin, Nigeria , Communication In Physical Sciences: Vol. 11 No. 3 (2024): VOLUME 11 ISSUE 3
- Abiodun Rasheed Omokanye, Benefit Onu, A Multi-Source Analysis of Water Supply Challenges in Offa Local Government Area, Kwara State, Nigeria , Communication In Physical Sciences: Vol. 11 No. 2 (2024): VOLUME 11 ISSUE 2
- Obialo Solomon Onwuka, Elochukwu Pearl Echezona, Chimankpam Kenneth Ezugwu, Hydrogeology of Uburu and Environs, Southern Eastern, Nigeria , Communication In Physical Sciences: Vol. 3 No. 1 (2018): VOLUME 3 ISSUE 1
- Henry Ekene Ohaegbuchu, Obinna Christian Dinneya, Chukwunenyoke Amos-Uhegbu, Paul Igienekpeme Aigba, Groundwater quality index (GQI) assessment of 12 wells in a rural area , Communication In Physical Sciences: Vol. 10 No. 2 (2023): VOLUME 10 ISSUE 2
- Ibrahim Usman, Williams Nashuka Kaigama, Thankgod Daniel, Abu Emmanuel Benjamin, Assessment of Water Quality from Hand Dug Wells in Kurmin Siddi, Kaduna State , Communication In Physical Sciences: Vol. 5 No. 2 (2020): VOLUME 5 ISSUE 2
- Chukwunenyoke Amos-Uhegbu, Mmaduabuchi Uche Uzoegbu, Okwuchukwu Peter Odoh , Chukwudike Dandy Akoma , Hydrogeology And Ground Water Potentials Of The Pre-Cambrian Basement Rocks Of Tabe And Environs In Gwagwalada Area, Abuja North Central, Nigeria , Communication In Physical Sciences: Vol. 10 No. 1 (2023): VOLUME 10 ISSUE 1
- Juliet E. Emudianughe, P. M. Eze, Sunday Utah, Porosity And Permeability Trend In Agbami-Field Using Well Log, Offshore, Niger Delta , Communication In Physical Sciences: Vol. 7 No. 4 (2021): VOLUME 7 ISSUE 4
- Usman Mohammed Ibrahim, Agada Livinus Emeka, Impacts of Climate Change on Groundwater Resources in a Semi-Arid Region: A Case Study of Damaturu, Yobe State , Communication In Physical Sciences: Vol. 11 No. 3 (2024): VOLUME 11 ISSUE 3
- Uba Sani, Abdulkadir Ibrahim, Akande, Esther Oluwatoyosi, John, Oghenetega Mercy, Murtala, Mohammed Rumah, Assessment of Surface Water Quality in Zaria Metropolis: Implications for Environmental Health and Sustainable Management , Communication In Physical Sciences: Vol. 11 No. 3 (2024): VOLUME 11 ISSUE 3
- Chidumebi Uzoho, The Role of Contaminated Water in Food Poisoning: An Assessment of Agricultural and Processing Practices , Communication In Physical Sciences: Vol. 12 No. 3 (2025): VOLUME 12 ISSUE 3
You may also start an advanced similarity search for this article.